Defect detection of gear parts in virtual manufacturing, Visual Computing for Industry, Biomedicine, and Art
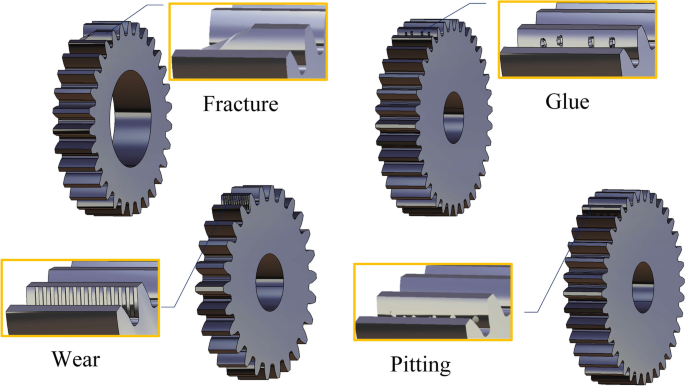
Gears play an important role in virtual manufacturing systems for digital twins; however, the image of gear tooth defects is difficult to acquire owing to its non-convex shape. In this study, a deep learning network is proposed to detect gear defects based on their point cloud representation. This approach mainly consists of three steps: (1) Various types of gear defects are classified into four cases (fracture, pitting, glue, and wear); A 3D gear dataset was constructed with 10000 instances following the aforementioned classification. (2) Gear-PCNet+ + introduces a novel Combinational Convolution Block, proposed based on the gear dataset for gear defect detection to effectively extract the local gear information and identify its complex topology; (3) Compared with other methods, experiments show that this method can achieve better recognition results for gear defects with higher efficiency and practicability.
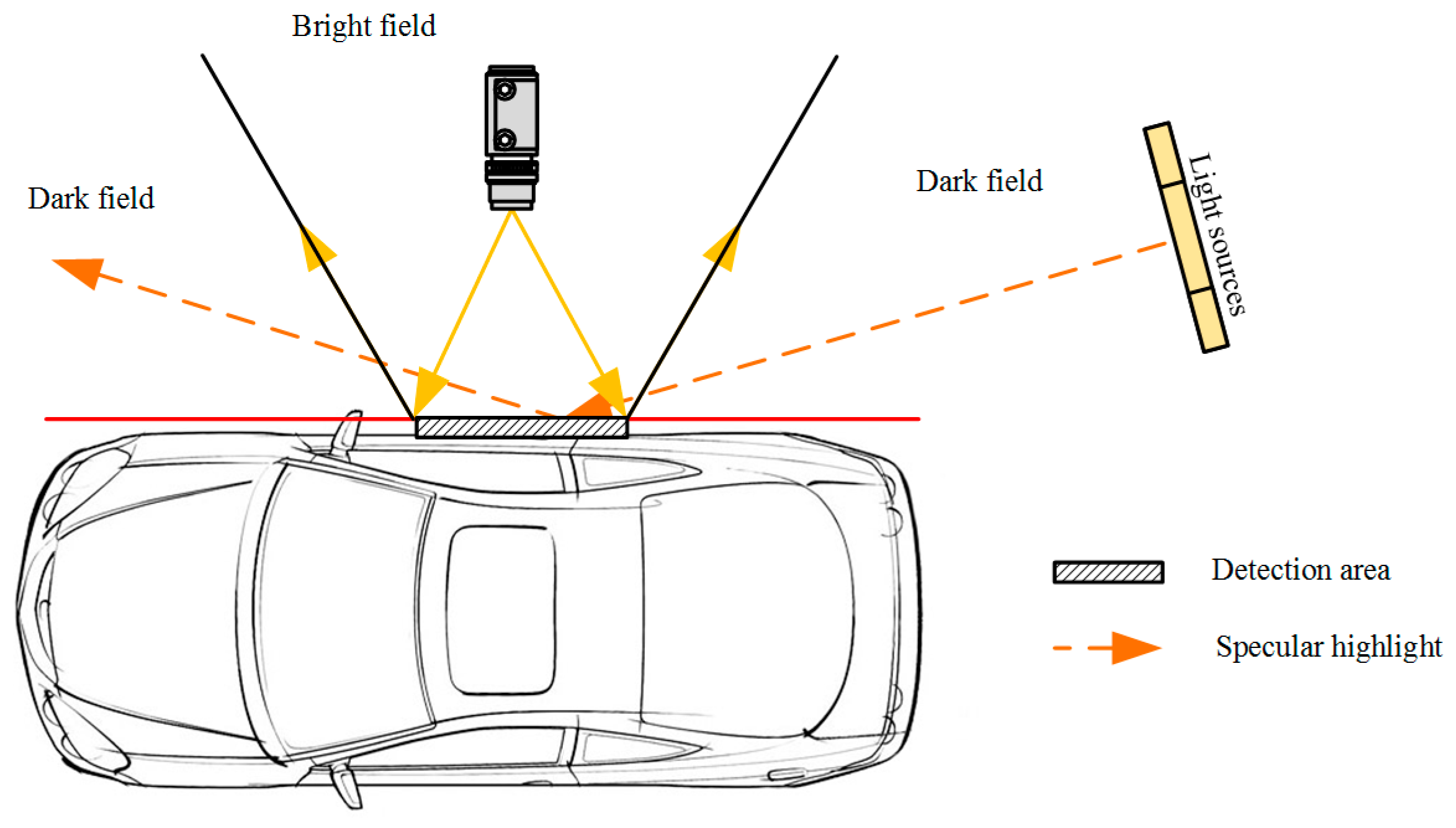
Sensors, Free Full-Text
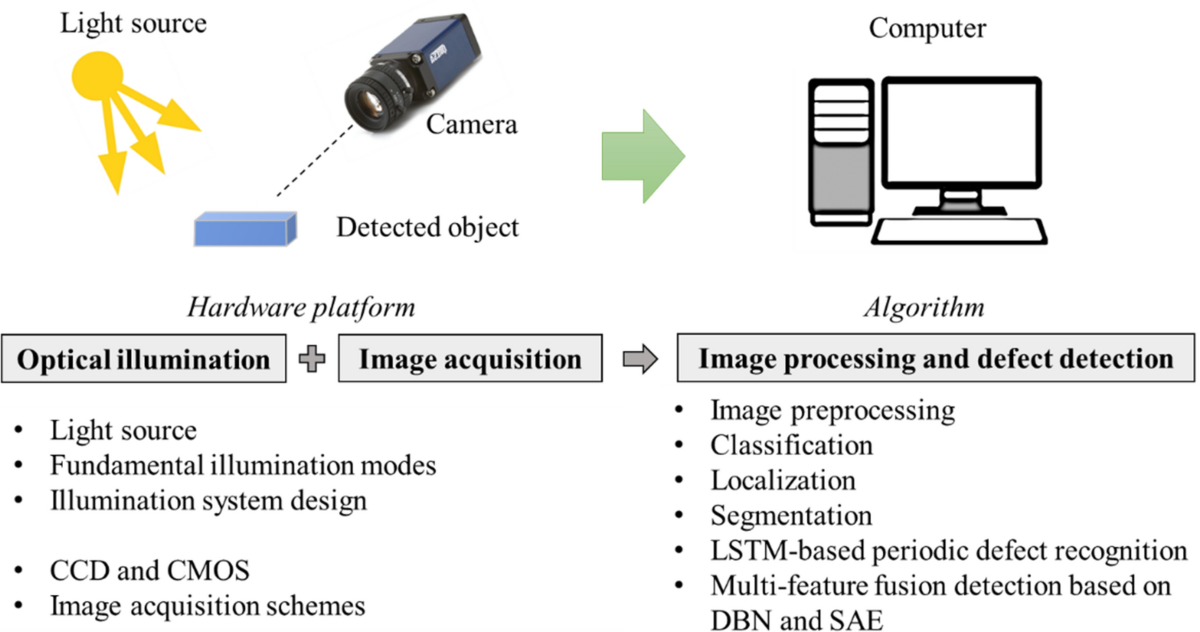
State of the Art in Defect Detection Based on Machine Vision
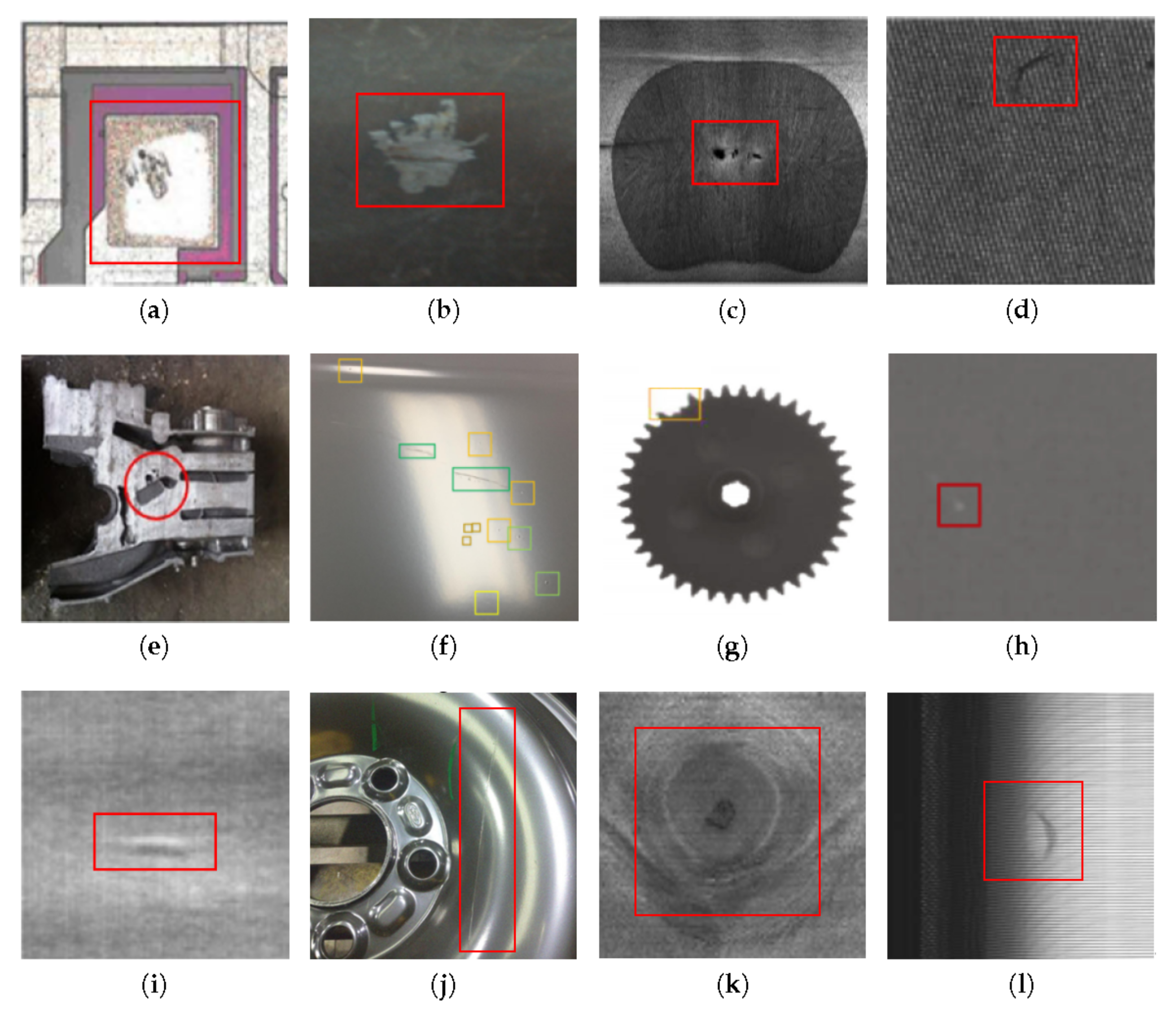
Materials, Free Full-Text
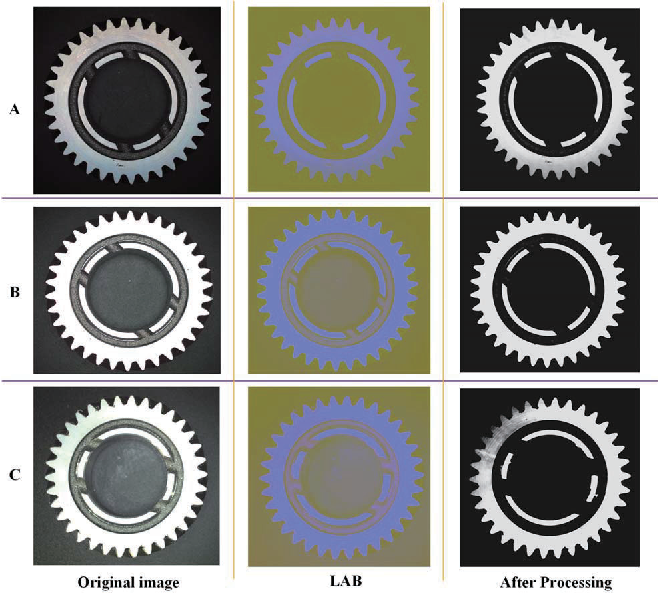
A defect detection method of gear end-face based on modified YOLO
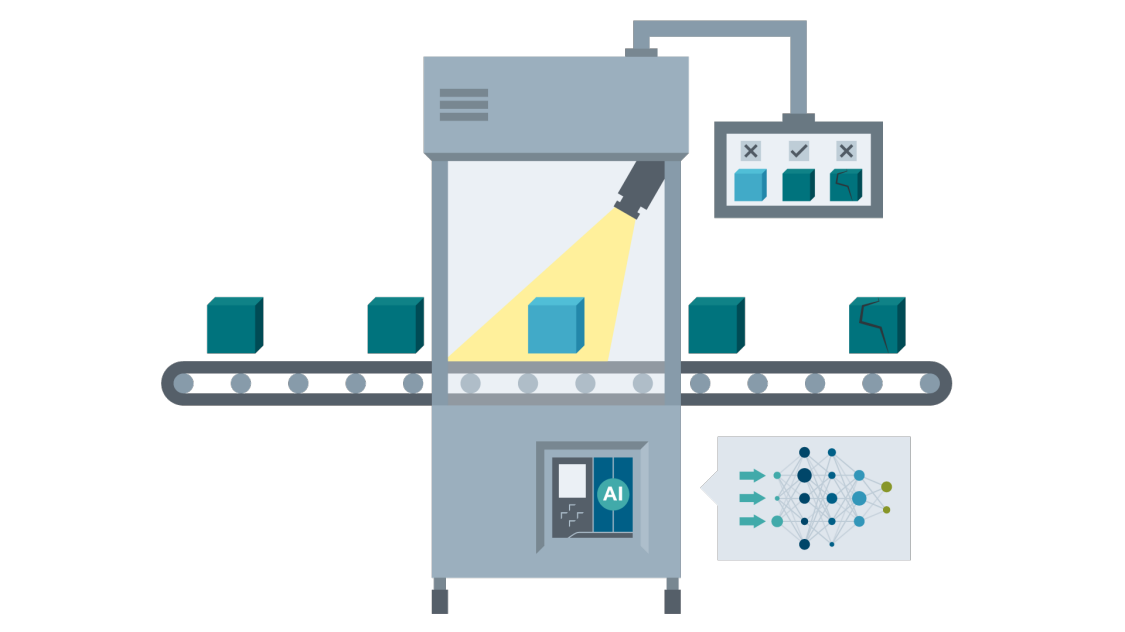
Vision Defect Detection Systems
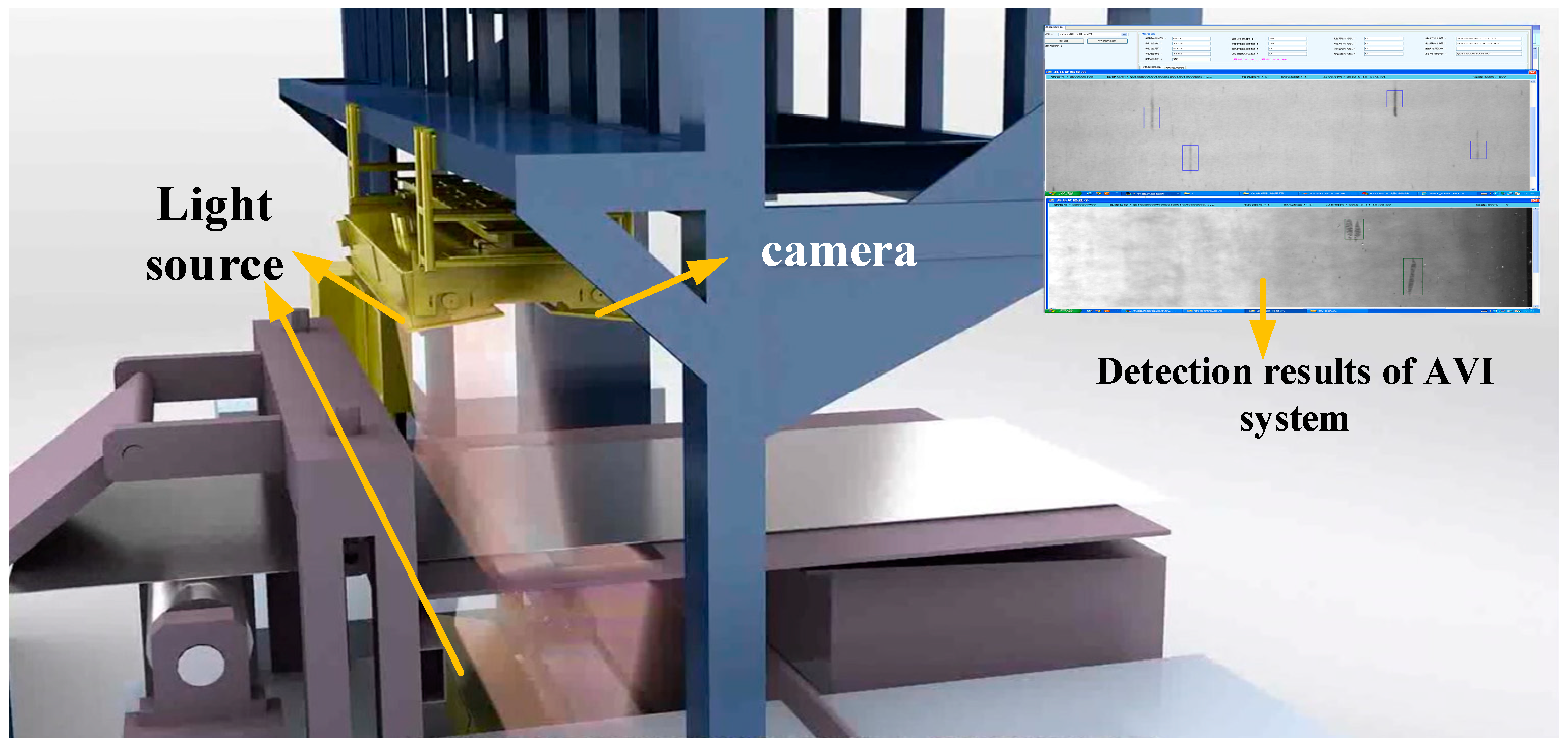
Sensors, Free Full-Text
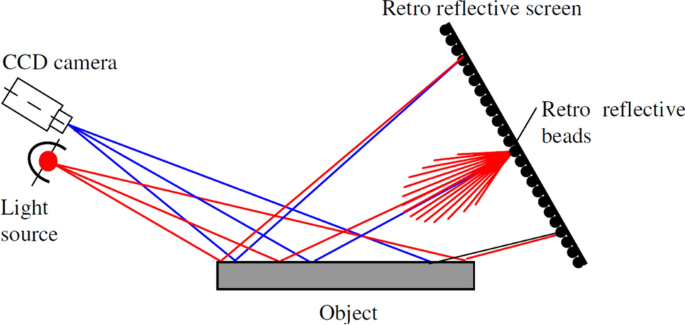
State of the Art in Defect Detection Based on Machine Vision
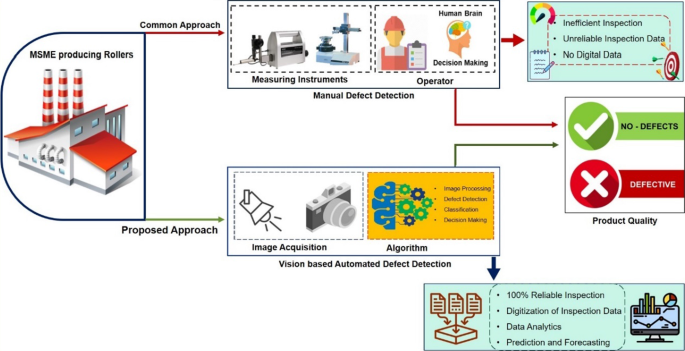
Automated surface defect detection framework using machine vision
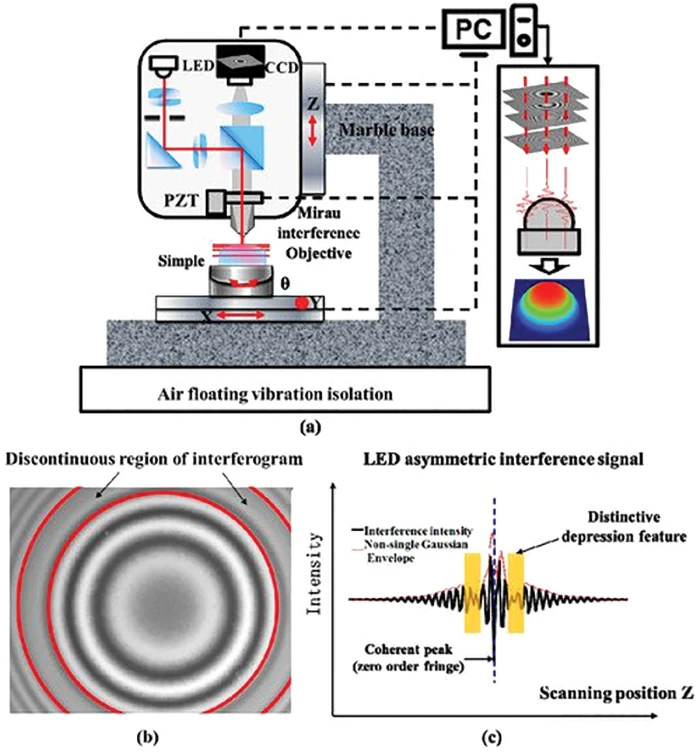
CMES, Free Full-Text
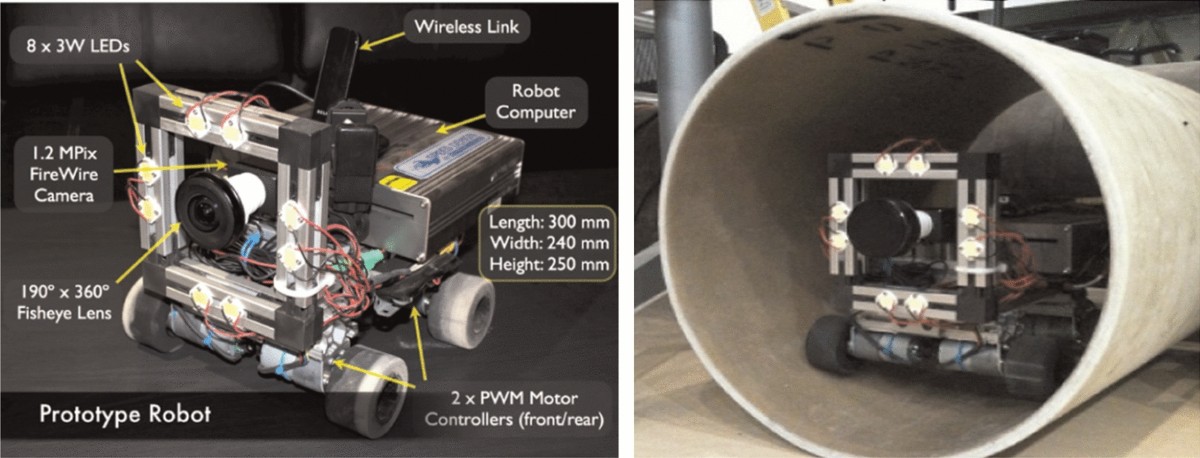
State of the Art in Defect Detection Based on Machine Vision
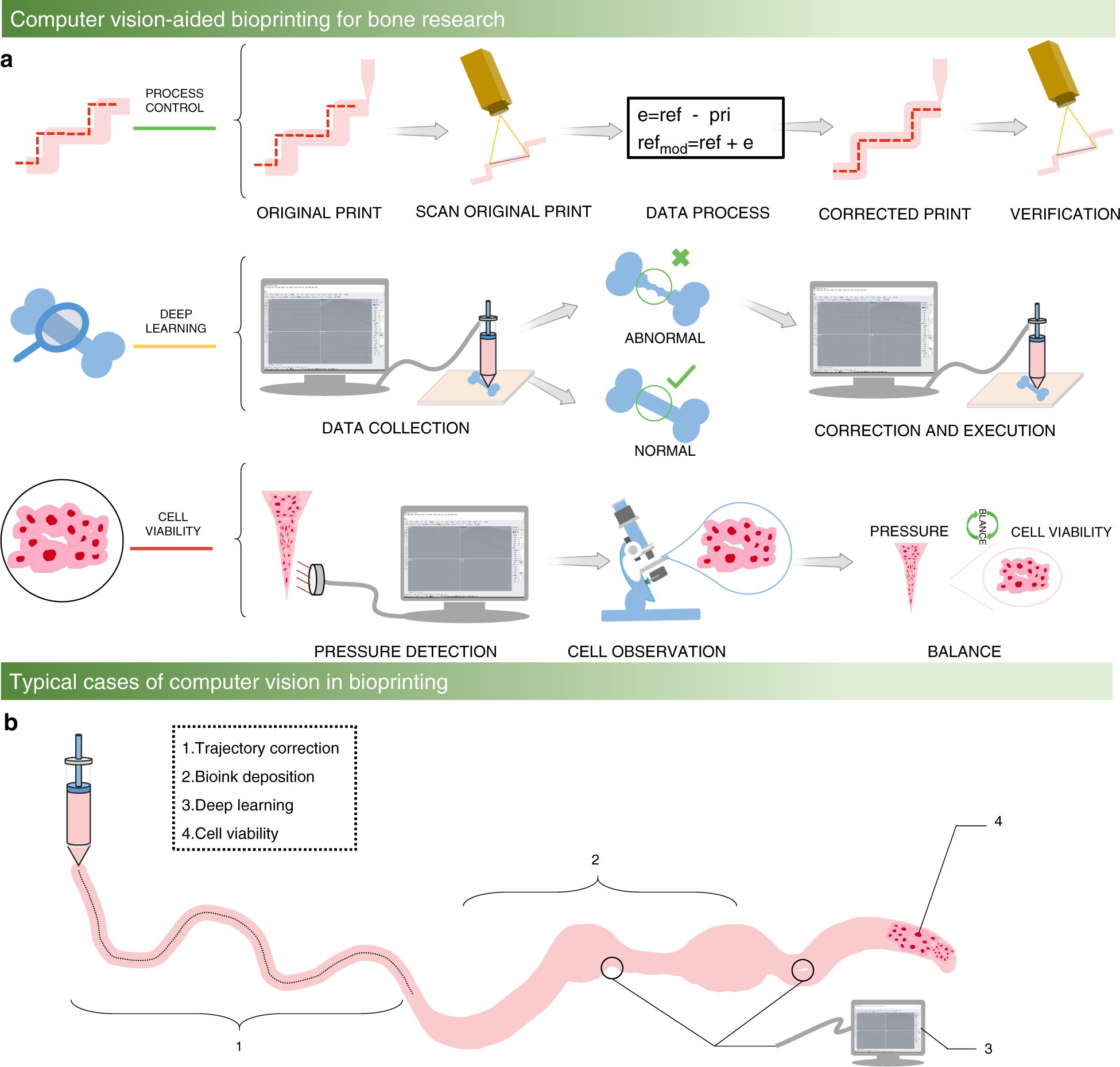
Computer vision-aided bioprinting for bone research

Journal of Mechanical Engineering 2012 7-8 by Darko Svetak - Issuu

Congenital parietal calvarial defects
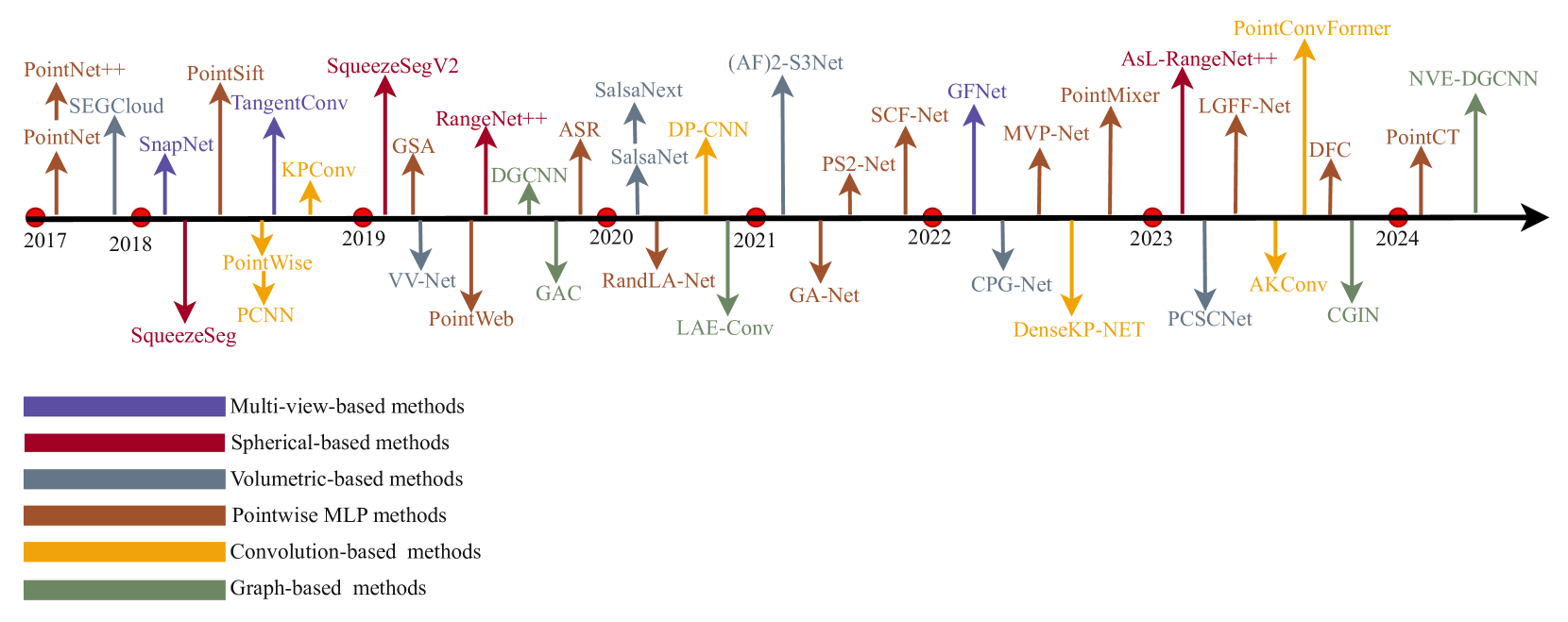
Advancements in Point Cloud-Based 3D Defect Detection and
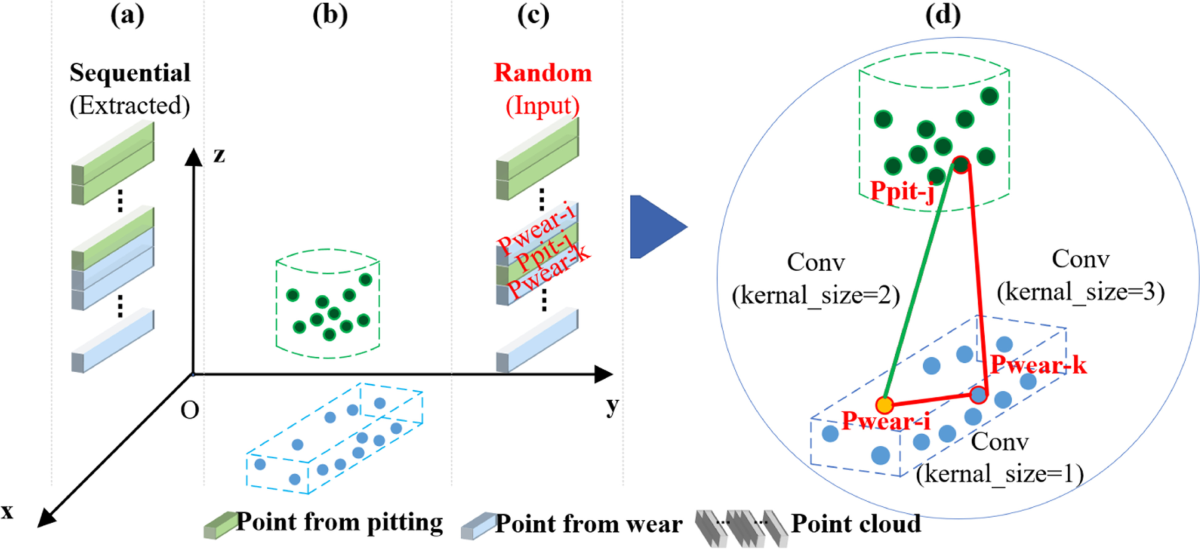
Defect detection of gear parts in virtual manufacturing